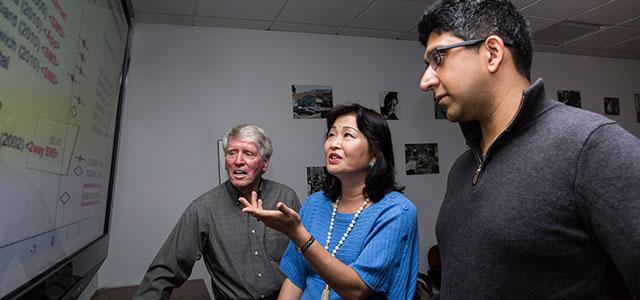
Yoshimi Fukuoka with William Haskell (left) and Anil Aswani (photos by Elisabeth Fall)
Big Data, mHealth and the Pursuit of Precision Behavior Change
Forget the miracle drugs. As many frontline clinicians understand, behavior change may be health care’s true Holy Grail.
The thinking is simple. Many chronic illnesses that plague individuals and drive up health care costs – such as diabetes, heart disease and some types of cancer – are preventable or more manageable if people change and maintain their diets and exercise regimens.
But behavior change is hard – particularly long-term behavior change.
“Numerous studies have shown that people struggle to sustain change for more than six months,” says Yoshimi Fukuoka from UC San Francisco School of Nursing’s Department of Physiological Nursing and Institute for Health & Aging. She says there are proven interventions that can get people past the six-month hurdle, but they tend to be costly and labor-intensive – not ideal or even practical at a time when the system is seeking ways to make health care less expensive and more efficient.
That’s why Fukuoka and others – inspired most recently by the 2015 federal Precision Medicine Initiative – have turned their attention to the vast potential in mobile health (mHealth). Their projects try to cost-effectively foster health-related behavior change through the use of mobile devices, such as physical activity trackers and mobile apps on smartphones.
In theory, mHealth connects individuals more frequently with their treatment goals while decreasing the need for face-to-face contact. It does this by generating a steady stream of targeted reminders and encouragement to prompt and sustain behavior change, while alerting providers when patients slip outside of prescribed regimens.
Fukuoka proposes that the right evidence-based apps could continuously update diet and exercise regimens based on objectively gathered data, rather than on self-reports, which are notoriously unreliable. The result would be a type of “precision behavior change” that might significantly improve outcomes and quality of life for vast numbers of people, while enhancing prevention for many more.
Technology Informed by Clinical Expertise
Fukuoka is working with two long-time collaborators – physical activity expert William Haskell from the Stanford Center on Longevity and health informatics expert Anil Aswani from UC Berkeley’s Industrial Engineering and Operations Research (IEOR) department – to develop and test a new generation of mHealth interventions that build on two successful studies Fukuoka completed in the past year.
Her mDPP (Mobile Based Diabetes Prevention Program) trial tested the ability of a mobile phone technology intervention to improve fasting plasma glucose levels and body weight in an overweight population at risk for type 2 diabetes. The approach was based on the Diabetes Prevention Program (DPP) trial, which found that lifestyle changes could effectively prevent or delay the onset of diabetes.
The original DPP intervention required 16 individual in-person sessions over 24 weeks. Fukuoka’s mDPP trial successfully reduced these individual in-person sessions to six – thus reducing the cost of the intervention – while achieving a similar effect on weight loss and other health outcomes. Fukuoka published her results in the American Journal of Preventive Medicine.
The second study – mPED (Mobile Phone Based Physical Activity Education) – showed that a mobile phone and pedometer-based intervention could help sedentary women meet widely accepted physical activity recommendations. The five-year clinical trial, funded by the National Heart, Lung, and Blood Institute (NHLBI), used a phone application to deliver the intervention, set individualized weekly goals and provide self-monitoring, immediate feedback and social support, while also communicating with researchers and providing real-time data collection.
“We also compared two different six-month maintenance interventions, with and without the app,” says Fukuoka. “This study is one of the first clinical trials to not only test the efficacy of the app itself, but also to evaluate the app-based maintenance strategy.”
Advancing the Science of mHealth
“One of the most interesting things to me is that often people only need very minor long-term changes to make a difference in their health,” says Fukuoka.
“Yoshimi’s work is extremely important in terms of moving this type of research and clinical practice agenda ahead. She’s been innovative in developing behavior change strategies that work well with smartphones and accelerometers,” says Haskell, who has served as a consultant and sounding board on many of her grants. He previously chaired the physical activity guidelines advisory committee for the Department of Health and Human Services, which issued its last set of guidelines in 2008. New guidelines are due in 2018.
Given the human and financial costs of cardiovascular disease and diabetes, Haskell believes Fukuoka’s work on the prevention of chronic illnesses is especially promising. “If we’re willing to put a little money up front to develop and evaluate these programs, they could deliver excellent return on investment,” Haskell says. “But there still is a lot of work needed to further develop, evaluate and modify these apps, so we can end up with an approach acceptable over the long term to a reasonable percentage of these patients.”
“There are a lot of physical activity and weight loss apps already available, but most are untested or not scientifically tested, and they often go unused, which is why our mDPP trial got so much attention,” says Fukuoka. “Now we want to advance the work based on behavior change science and sophisticated algorithms that enable us to adapt our interventions to individual personalities in culturally sensitive ways. In our preliminary work, we found this type of gradual, adapted encouragement seems to make a difference.”
Thus in this new project, funded by the National Science Foundation and the National Institute of Nursing Research, she and Aswani will use big data in real time for what she calls a “behavioral-adaptive” clinical trial that tests whether a large, culturally diverse prediabetic population can sustain the behavior change needed to ward off diabetes onset.
Can Big Data Personalize Care?
It’s Aswani’s task to convert Fukuoka’s psychological understanding of health care decisionmaking and behavior change into the mathematical models and equations that serve as the back end of any application.
“If we give someone a goal for how many steps to take, we need to quantify how much satisfaction the person derives for getting close to the goal,” says Aswani, who has long had a passionate interest in using data to personalize health. “We use this quantification – which is estimated from a profile that is dynamically constructed based on a survey participants fill out at the beginning of the trial, along with objective data we gather about physical activity – in order to predict current and future motivational states. Such a quantitative understanding of motivational states can be used to individualize the intervention.”
The team has already developed the algorithms, filed a patent application and submitted a paper that describes their quantitative modeling approach. They are now working on a second paper, which describes how to use the models to optimally select and individualize goals and schedules of visits. They hope to have a clinical trial up and running in the fall of 2016 that will focus first on physical activity alone. As they test and refine their intervention, they expect to expand it to weight loss.
“We believe that by adding adaptation, people should be able to do better, and we’re curious how much better they can do,” says Aswani. “Our initial simulations are promising.”
Fukuoka’s team is using a similar approach to take her mPED trial to the next step as well, seeking to sustainably increase the physical activity of previously sedentary women, middle-aged to older.
“We want to design a personalized intervention that can engage people throughout the day, because we know that frequently getting up from sitting has positive effects, but many people only designate exercise for a specific time of the day,” she says.
“The study is primarily focused on older women, with very substantial race and ethnic diversity, which is really important,” says Haskell. “She’s targeting populations that need these interventions, and that’s why organizations like the NIH [National Institutes of Health] and American Heart Association are interested.”
Much More Work to Do
Haskell is quick to add, however, that there is much more work to do.
“It’s correct to be positive about the results of these early studies but wise to be conservative,” he says. “There’s no guarantee that the new technologies and analytics will result in a significant, long-term change in people at risk. As of today we have very little solid evidence.”
One of the major limitations, he says, is that the software engineers are ahead of the psychologists and behavioral change experts, who are still trying to validate what works to sustain behavior change, especially in high-risk individuals and those from underserved communities with limited access to health care. Nevertheless, the team feels what they are doing has the potential to address these types of concerns.
“Gathering all of this data can give us a better understanding of what people value, and we can use that to help them with their programs,” says Aswani.
He notes, for example, that many people don’t necessarily make their long-term health a high priority, so these types of interventions can address the more immediate motivations while also coaching people to understand why long-term health matters.
“One of the most interesting things to me is that often people only need very minor long-term changes to make a difference in their health,” says Fukuoka. “So much depends on education, and we believe these apps can educate people.”