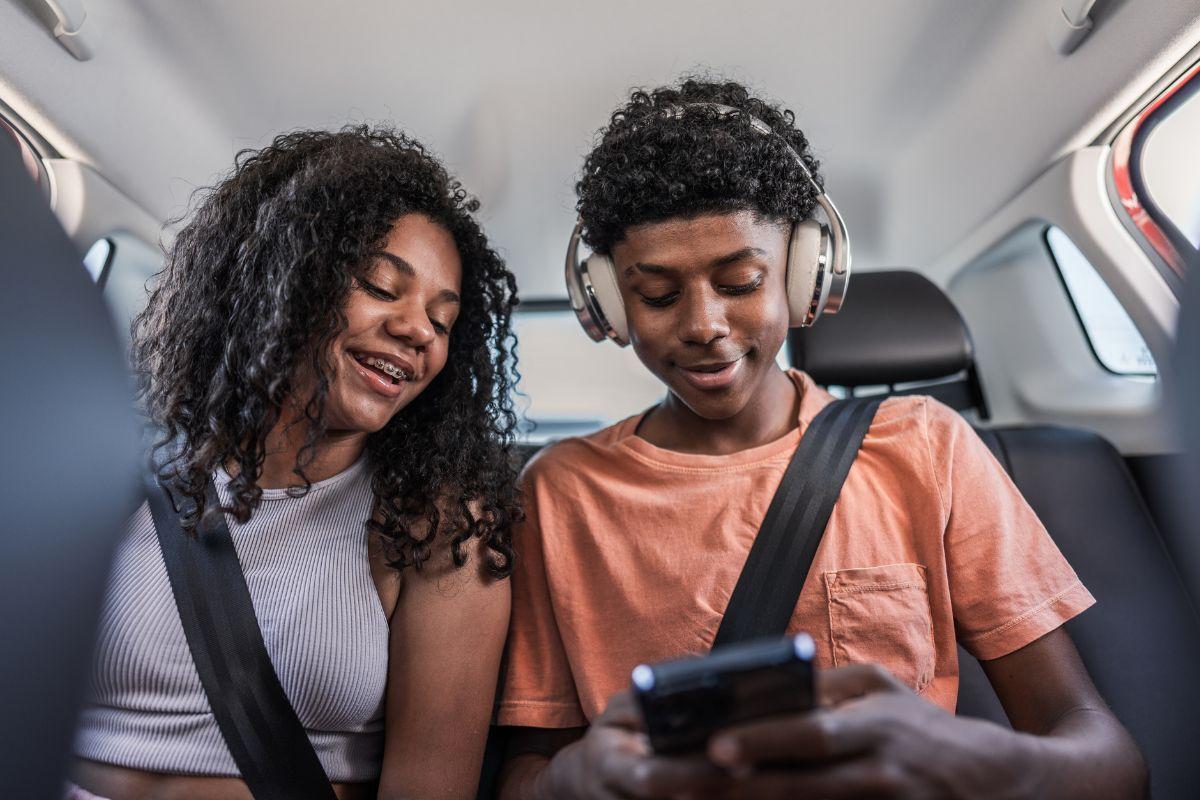
Innovating Wellness: Health Research and Artificial Intelligence
Artificial intelligence (AI) and machine learning (ML) are emerging technologies with the potential to revolutionize health care, using powerful algorithms to do everything from make health care more efficient to find new cures for disease. But the rapid advancement of technology in health care demands caution. Health researchers must apply technologies effectively for the right use cases and ethically, to accelerate versus hamper equitable patient care.
Experts in the UCSF School of Nursing are leading innovative projects that harness the power of AI to advance health equity and improve health — but doing so responsibly, making sure the right projects, for the right reasons, are undertaken with ethical care.
Ethical Dimensions of Utilizing Innovative and Artificial Intelligence Technologies in Health Care
Those ethics are top of mind for Anita Ho, PhD, MPH, associate professor in the School of Nursing, faculty in the UCSF Bioethics program and author of “Live Like Nobody is Watching: Relational Autonomy in the age of Artificial Intelligence Health Monitoring.”
“One of the things I worry about, as we try to be quick in developing and implementing AI algorithms, is whether they’re actually accurate for all the populations that we’re serving,” she said.
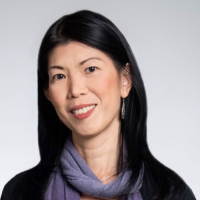
That means looking at the work behind some of the successes being touted by AI models, especially if that work is shrouded from view. “Sometimes we have models that seem promising, but they often are only piloted or tested on very small populations,” she said. That’s especially a concern for so-called “black box” algorithms developed by private companies that don’t share information about their algorithms, or even the source of their data. This also makes their models impossible to validate by testing them independently.
If these algorithms are sold to people as direct-to-consumer products, which do not have to meet rigorous standards, or results are given without proper interpretation by medical professionals, then people could be operating with shaky data when making important health care decisions.
Ho also wants researchers and health care providers to think through how AI can be best used as opposed to applying it everywhere and hoping it’s effective. For example, AI could help clinics determine which patients are most likely to not show up to an appointment. But instead of double booking those appointment slots on the assumption that a patient is not going to keep it, resources could be applied to help that person overcome the barriers that might be preventing them from coming into the clinic. Not only will that help patients navigate challenges like childcare and transportation, it also avoids punishing them with longer wait times if they are able to attend.
“They may think ‘why did I even bother coming in the first place?’” Ho said. “AI algorithms should actually serve the patients who are most vulnerable, even if they are serving the health system in the broader sense.”
Optimizing AI Voice to Effectively Disseminate Information to Teens and Parents
With the rise in popularity of podcasting and audiobooks, delivering content through your ears has become a more accepted, even preferred, way to receive and digest information.
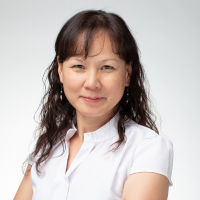
“As voice becomes very popular, what that voice sounds like matters,” said Jyu-Lin Chen, PhD, RN, FAAN, professor. “There is a big social impression even if you hear only the voice.”
That means that in AI-powered resources, we must consider the sound of the voice. Through a grant from the U.S. Centers for Disease Control and Prevention, Chen was tasked with taking text-based modules on general health communication, sexual health and reproductive communication, parental monitor and well-child checkups, and seeing which voice would be best received by listeners in each context.
The research team asked both teenagers and their parents/guardians to rate eight different voices on things like intelligibility, naturalness, social impression, trustworthiness, and overall appeal and prosody, which is rhythm and meter. Chen thought that each group would ultimately select different kinds of voices out of the eight offered. Instead, both groups preferred mature, female voices.
The study was only conducted on 104 people, but shows the importance of trying different voices for people across demographics, so that they are most likely to receive critical, health-related information.
“Just like with GPS, you can have different GPS voices. What attracts you to that voice most? What do you like to hear?” she asked.
Using Machine Learning Approaches to Develop and Evaluate Models for Predicting Symptoms in Oncology Patients
Trying to determine which patients will have what side effects from chemotherapy can be tricky. And while chemotherapy’s goal is to combat cancer and alleviate its symptoms, the treatment’s side effects can also gravely impact patients, plummeting their quality of life and affecting overall outcomes.
That’s why Kord Kober, PhD, associate professor, has been working on machine learning-enabled algorithms that can predict who is most at risk for developing side effects, and how severe those symptoms may be. “These models could assist clinicians in identifying high-risk patients and provide them with recommendations for modifying activities and interventions to manage or reduce these symptoms,” he said.
The research team decided to use machine learning because of its “focus on the development of the best predictive model,” he said. Plus, machine learning “can extract large amounts of data from a relatively small number of samples.”
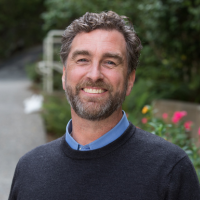
For example, with a sample of 1,217 patients, researchers looked at 157 different demographic, clinical, symptom and psychological factors to determine which patients would be most likely to develop fatigue because of chemotherapy, and how serious it might be. They found that regardless of other factors, simply asking patients to rate their levels of feeling “worn out” or “exhausted” on a scale of 1 to 10 was predictive.
While promising, these models are not yet used in clinical practice — nor should they be just yet. Several steps need to happen first, said Kober, including that the models be independently verified with different groups of patients, and have an evaluation of their trustworthiness via Health and Human Services’ Trustworthy AI guidelines, which have been adopted by UCSF.
“This model was a first step and there is plenty of work to do before we can get it into the clinic,” he said.
He is also keenly aware of how bias can slide into machine learning models, and the importance of doing things like testing these models on wide groups of people and making sure that AI is not just a “set it and forget it” application, but is continually monitored. “These issues must be addressed in all prediction models and are paramount for the models already being deployed in the clinic,” Kober said.